The fusion of artificial intelligence (AI) and low code development stands at the forefront of technological advancement. Now, the complex software applications can be created with minimal coding expertise, where the power of AI supplements human creativity, and where ethical considerations drive every aspect of technological progress. The integration of AI integration in low code development holds the promise of revolutionizing how software is built, deployed, and managed.
We have already stepped into the world of AI and this is meant to co-exist with us.
While we may set the initial parameters and provide the data, AI often takes the reins, exploring avenues and solutions that might never have occurred to us. This element of autonomy and self-directed learning is what sets AI apart, pushing the boundaries of what we thought possible and challenging us to reimagine the role of technology in our lives.
But with great power comes great responsibility. The integration of AI is not free of its limitation. It is fraught with ethical challenges and moral dilemmas.
So the matter of concern is how we ensure fairness and equity in AI-powered decision-making.
How do we maintain transparency and accountability in algorithms that operate behind the scenes?
How do we safeguard the privacy and security of individuals in an increasingly interconnected world?
These are the questions that demand our attention as we read more about AI integration in low code development.
The Current Landscape of AI Integration in Low Code
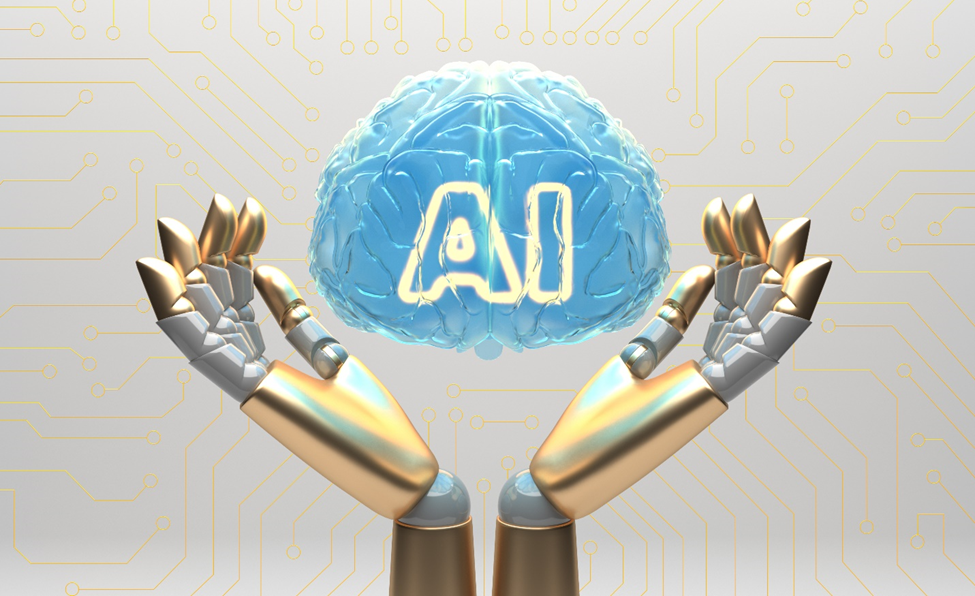
Low code development platforms have emerged as game-changers in the software industry, empowering businesses and individuals to create applications with unprecedented speed and efficiency.
From drag-and-drop interfaces to visual modeling tools, low code platforms democratize the process of software development, enabling even non-technical users to participate in the creation of digital solutions. And now, with the integration of AI capabilities, these platforms are poised to unlock new levels of innovation and productivity.
AI integration in low code development encompasses a wide range of functionalities, from natural language processing for automating repetitive tasks to machine learning (ML) algorithms for predictive analytics.
These AI-powered features not only streamline the development process but also enhance the intelligence of applications, enabling them to adapt and evolve in response to changing user needs and market dynamics. Whether it is automating business workflows, optimizing resource allocation, or personalizing user experiences, AI integration in low code opens up a world of possibilities for innovation and growth.
Examples of AI integration in low code tools abound, from Chabot builders that leverage NLP to create conversational interfaces to predictive modeling platforms that utilize ML to generate insights from data. These tools empower developers to make the most of AI without requiring deep expertise in machine learning or data science, democratizing access to advanced technology and accelerating the pace of digital transformation.
Ethical Challenges in AI Integration
1. Bias and Fairness Issues:
One of the foremost ethical challenges in AI integration is the presence of bias and fairness issues. AI systems are only as unbiased as the data they are trained on, and if that data contains biases, the resulting algorithms will perpetuate and potentially amplify those biases. This can lead to discriminatory outcomes, particularly in sensitive areas such as hiring, lending, and law enforcement.
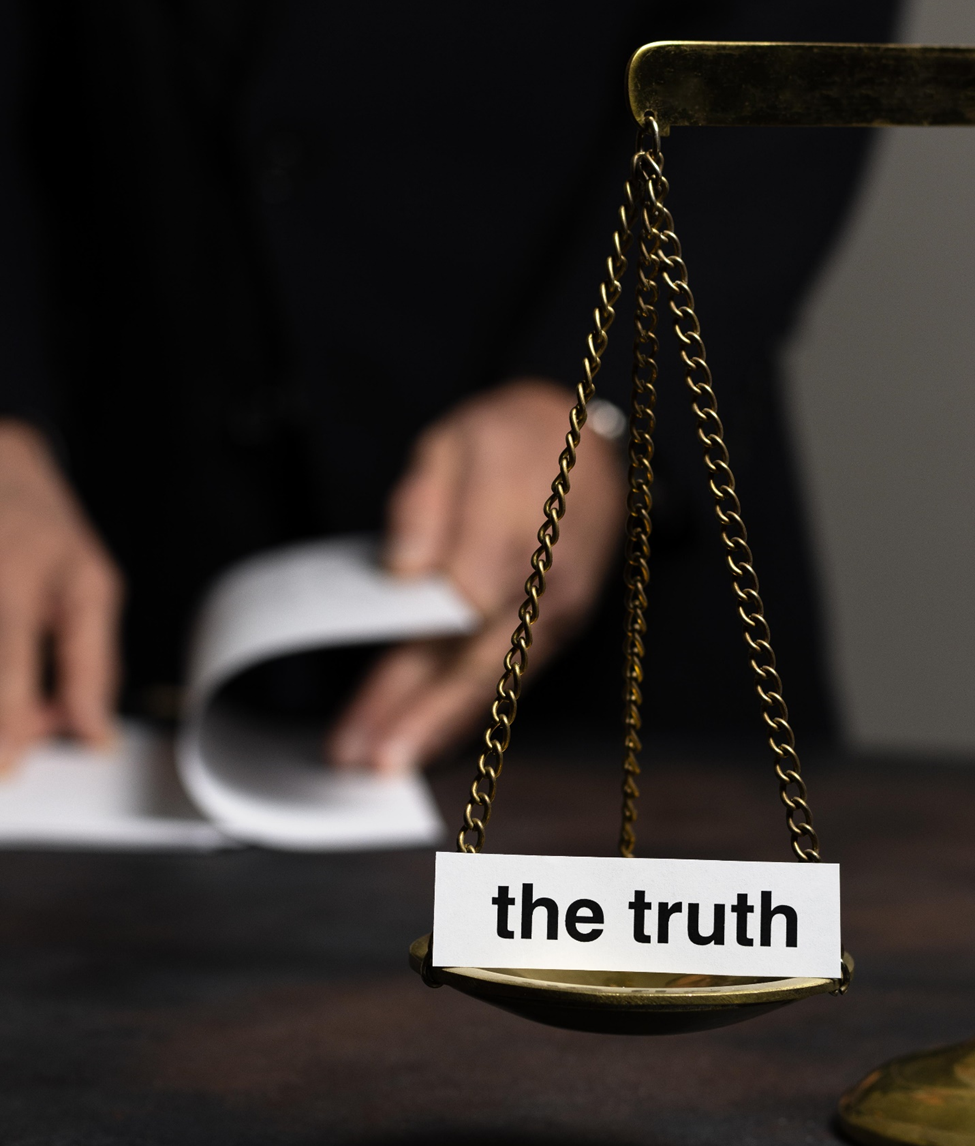
Addressing bias requires careful consideration at every stage of the AI development lifecycle, from data collection and preprocessing to algorithm design and deployment. It also necessitates diverse and inclusive teams to identify and mitigate biases effectively.
2. Transparency:
Transparency is essential for building trust in AI systems, yet they often pose significant challenges. Many AI algorithms, especially those based on deep learning techniques, are notoriously opaque, making it difficult to understand how they arrive at their decisions.
The lack of transparency can erode trust and accountability, particularly in high-stakes applications such as healthcare and criminal justice.
To address this challenge, researchers are developing methods for explaining AI decisions, such as interpretable models and algorithmic auditing techniques. However, achieving true transparency remains an ongoing area of research and development.
3. Privacy Concerns:
AI integration raises profound privacy concerns, particularly regarding the collection and use of personal data. AI systems often rely on large datasets to train and improve their performance, raising questions about consent, data ownership, and individual privacy rights.
The increasing deployment of AI in surveillance and data-driven decision-making exacerbates these concerns, amplifying the potential for privacy violations and surveillance abuses. Striking a balance between innovation and privacy protection requires robust data governance frameworks, clear regulations, and mechanisms for obtaining informed consent from individuals whose data is being used.
4. Legal and Regulatory Compliance:
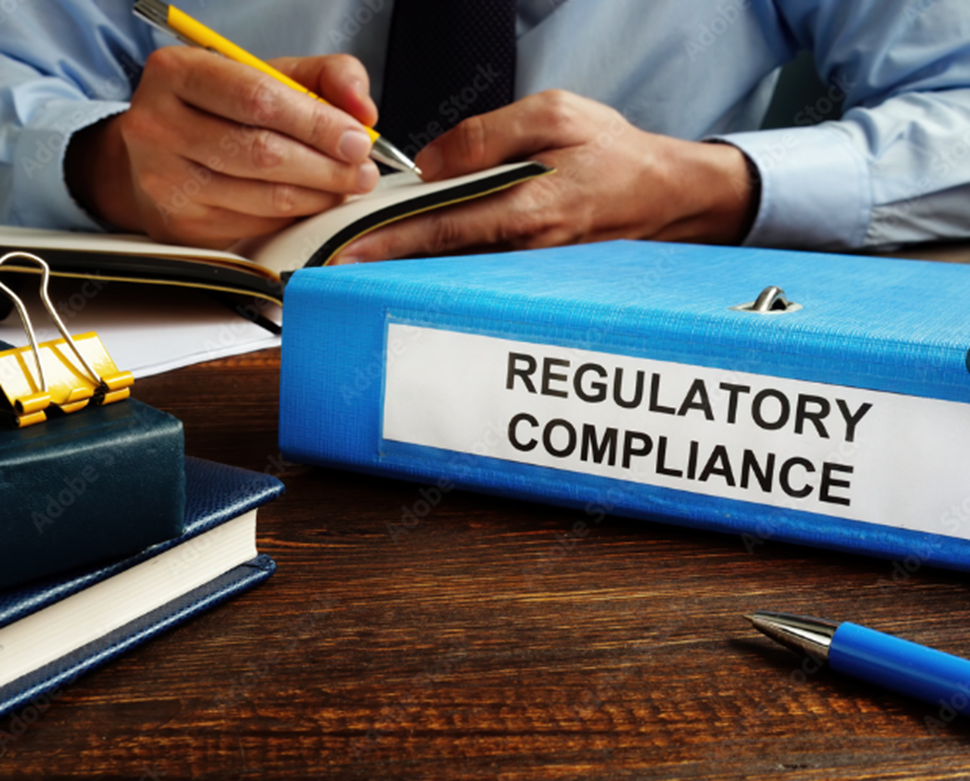
Navigating the legal and regulatory landscape surrounding AI integration is another significant ethical challenge. As AI technologies become more pervasive and influential, policymakers are grappling with how to regulate them effectively.
Questions of liability, accountability, and responsibility loom large, particularly in cases where AI systems cause harm or make consequential decisions.
Ensuring legal and regulatory compliance requires collaboration between policymakers, industry stakeholders, and civil society to develop clear guidelines, standards, and oversight mechanisms that protect individual rights and promote responsible AI deployment.
Ethical Progression Enabled by AI Integration
1. Automation of ethical checks
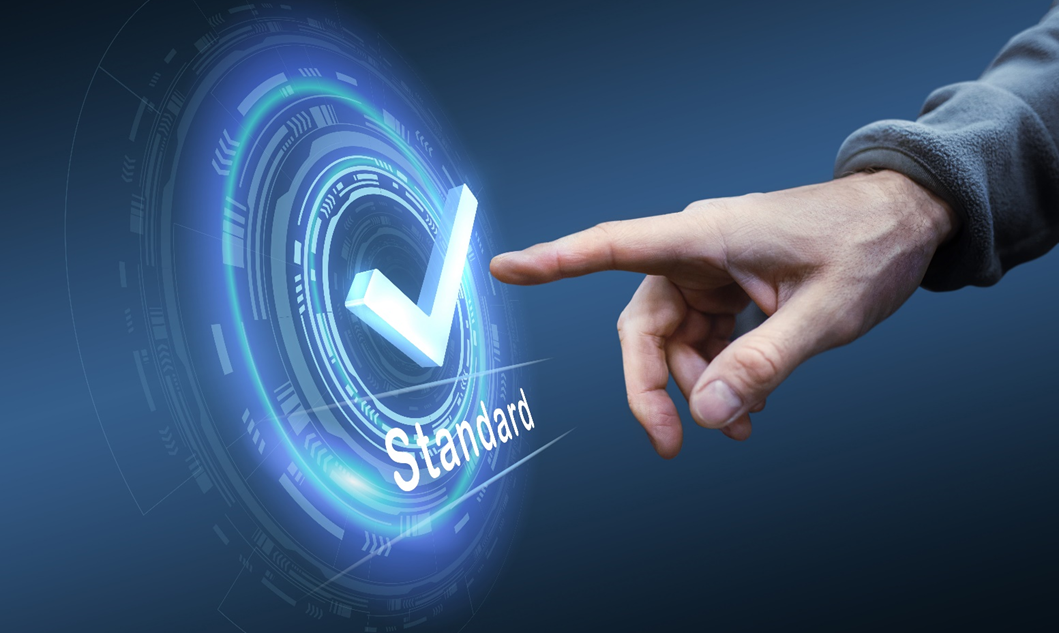
AI integration offers the potential to automate ethical checks and safeguards throughout the AI development lifecycle. For example, AI systems can be trained to detect and mitigate biases in datasets, ensuring fair and equitable outcomes.
Similarly, automated auditing tools can monitor AI systems in real-time to identify and address ethical issues as they arise. By using AI for ethical oversight, organizations can enhance transparency, accountability, and trust in their AI deployments.
2. Improved Accessibility and Inclusivity
AI integration in low code development can improve accessibility and inclusivity by democratizing access to advanced technology. Low code platforms enable individuals with diverse backgrounds and skill levels to participate in the creation of AI-powered solutions, fostering greater diversity of perspectives and expertise.
AI-driven accessibility features, such as natural language processing and assistive technologies, can empower individuals with disabilities to engage more fully in digital experiences. By prioritizing accessibility and inclusivity in AI integration efforts, organizations can create more equitable and user-centric solutions.
3. Enhanced Efficiency and Productivity
AI integration in low code development can enhance efficiency and productivity by automating repetitive tasks and streamlining workflows. AI-powered tools can assist developers in coding, debugging, and testing software, reducing the time and effort required to bring products to market.
AI-driven analytics and insights can inform decision-making and optimize resource allocation, driving greater efficiency and effectiveness across organizations. By harnessing AI to augment human capabilities, organizations can achieve higher levels of productivity and innovation in low code development.
4. Potential for Innovation in Ethical Design
AI integration opens up new opportunities for innovation in ethical design practices. By incorporating AI-driven techniques such as generative design and predictive modeling, developers can explore alternative solutions and anticipate potential ethical challenges before they arise.
AI-powered tools for ethical decision-making and impact assessment can guide developers in designing more responsible and sustainable AI systems. By embracing AI as a catalyst for ethical innovation, organizations can proactively address ethical considerations and create more resilient and trustworthy AI solutions.
Regression: Ethical Risks and Pitfalls
1. Amplification of Bias
Despite efforts to mitigate bias, AI integration can inadvertently amplify existing biases present in training data, leading to discriminatory outcomes and reinforcing systemic inequalities. This amplification of bias poses significant ethical risks, particularly in domains such as hiring, lending, and criminal justice, where AI-driven decisions can have far-reaching consequences.
Addressing this challenge requires ongoing vigilance and proactive measures to identify and mitigate biases at every stage of the AI development lifecycle.
2. Lack of Accountability
The opacity and complexity of AI systems can create challenges in establishing accountability for their decisions and actions. When AI systems produce erroneous or harmful outcomes, it may be difficult to attribute responsibility or liability, leading to a lack of accountability.
This lack of accountability undermines trust and confidence in AI technologies, potentially eroding public acceptance and hindering their adoption. Establishing clear lines of accountability, along with mechanisms for recourse and redress, is essential for ensuring responsible AI deployment and fostering trust among stakeholders.
3. Overreliance on AI Decision-Making
An overreliance on AI decision-making poses ethical risks, particularly when human judgment and oversight are sidelined or overridden. While AI can enhance decision-making by providing data-driven insights and predictions, it should not replace human judgment entirely.
Blindly following AI recommendations without critical evaluation can lead to erroneous or harmful outcomes, especially in complex and dynamic environments. Striking the right balance between AI assistance and human judgment is essential for ensuring ethical decision-making and safeguarding against unintended consequences.
4. Potential for Exploitation and Manipulation
The increasing sophistication of AI technologies raises concerns about their potential for exploitation and manipulation. Malicious actors may exploit vulnerabilities in AI systems to manipulate outcomes or deceive users for nefarious purposes.
The use of AI-driven misinformation and deepfake technologies can undermine trust in information and erode social cohesion. Combatting these threats requires a multi-faceted approach that combines technical safeguards, regulatory measures, and public awareness campaigns to detect and mitigate AI-driven manipulation effectively.
Mitigation Strategies and Best Practices
1. Incorporating Ethical Design Principles
Incorporating ethical design principles into AI integration efforts is essential for ensuring responsible and equitable outcomes. This involves prioritizing values such as fairness, transparency, accountability, and privacy throughout the AI development lifecycle.
Ethical design principles can guide decision-making at every stage, from data collection and preprocessing to algorithm design and deployment. For example, organizations can adopt frameworks such as the Fairness, Accountability, and Transparency (FAT) principles or the Ethical AI Guidelines developed by industry associations and regulatory bodies.
By embedding ethical considerations into the design process, organizations can proactively address potential ethical risks and promote trust and confidence in AI technologies.
2. Implementing Bias Detection and Mitigation Techniques
Detecting and mitigating biases in AI systems requires a multi-pronged approach that combines technical tools, domain expertise, and diverse perspectives. Organizations can implement bias detection and mitigation techniques at various stages of the AI development lifecycle, including data collection, preprocessing, model training, and evaluation.
Techniques such as data auditing, bias-aware algorithms, and adversarial testing can help identify and address biases in training data and algorithmic decision-making. Involving diverse stakeholders, including domain experts, ethicists, and affected communities, can provide valuable insights into potential biases and their implications.
By proactively addressing bias, organizations can enhance the fairness and equity of AI-driven solutions and mitigate the risk of discriminatory outcomes.
3. Enhancing Transparency and Accountability Mechanisms
Organizations can adopt practices such as algorithmic transparency and accountability by design to ensure that AI-driven decisions are understandable and auditable. This may involve providing explanations for AI decisions, disclosing the underlying algorithms and data sources, and establishing mechanisms for monitoring and auditing AI systems in real-time.
Companies can implement governance structures and oversight mechanisms to hold stakeholders accountable for the ethical use of AI technologies. By promoting transparency and accountability, organizations can foster greater trust and acceptance of AI-driven solutions among users, regulators, and society at large.
4. Strengthening Legal and Regulatory Frameworks
Strengthening legal and regulatory frameworks is important for addressing the ethical challenges associated with AI integration. Policymakers can develop clear guidelines, standards, and regulations that govern the development, deployment, and use of AI technologies. This may include measures such as data protection regulations, algorithmic accountability laws, and standards for AI ethics and governance.
Regulatory agencies can establish oversight mechanisms and enforcement mechanisms to ensure compliance with ethical principles and legal requirements. By strengthening legal and regulatory frameworks, policymakers can create an enabling environment for responsible AI innovation while safeguarding individual rights and promoting societal well-being.
Conclusion
In conclusion, AI integration in low code development holds immense promise for revolutionizing how software is built, deployed, and managed. However, realizing this potential requires understanding ethical challenges and considerations. From bias and fairness issues to transparency and accountability mechanisms, organizations must proactively address ethical concerns at every stage of the AI development lifecycle.
The future of AI integration in low code development is filled with both opportunities and challenges. As AI technologies continue to evolve and mature, organizations need to prioritize ethical considerations and adopt best practices to ensure responsible and equitable AI deployment.
By working together to address ethical challenges and promote responsible AI deployment, we can build a future where technology serves humanity with wisdom, compassion, and integrity. Let us seize this opportunity to shape a future where AI integration in low code development leads us towards ethical progression, not regression.